Ultimate Guide to AI Warehouse Safety
This guide provides insights and strategies for using artificial intelligence (AI) to make warehouses safer places to work. Whether you’re a warehouse manager or a health and safety professional, you’ll gain a greater understanding of how...
Enhancing warehouse safety with AI technology
We know warehouses can be dangerous places, and that managing the hazards takes a lot of effort and vigilance.
This guide provides insights and strategies for using artificial intelligence (AI) to make warehouses safer places to work by improving safety procedures and mitigating potential hazards.
Whether you’re a warehouse manager or a health and safety professional, you’ll gain a greater understanding of how emerging technologies can help you manage occupational safety challenges and compliance issues within warehouse operations.
This guide makes no assumptions of your current understanding of the technology. You might have existing safety issues you know you need to tackle. Or you might have a good system - but you’re looking for continuous improvement. Either way, this guide explains how using AI in warehouse safety management can help.\
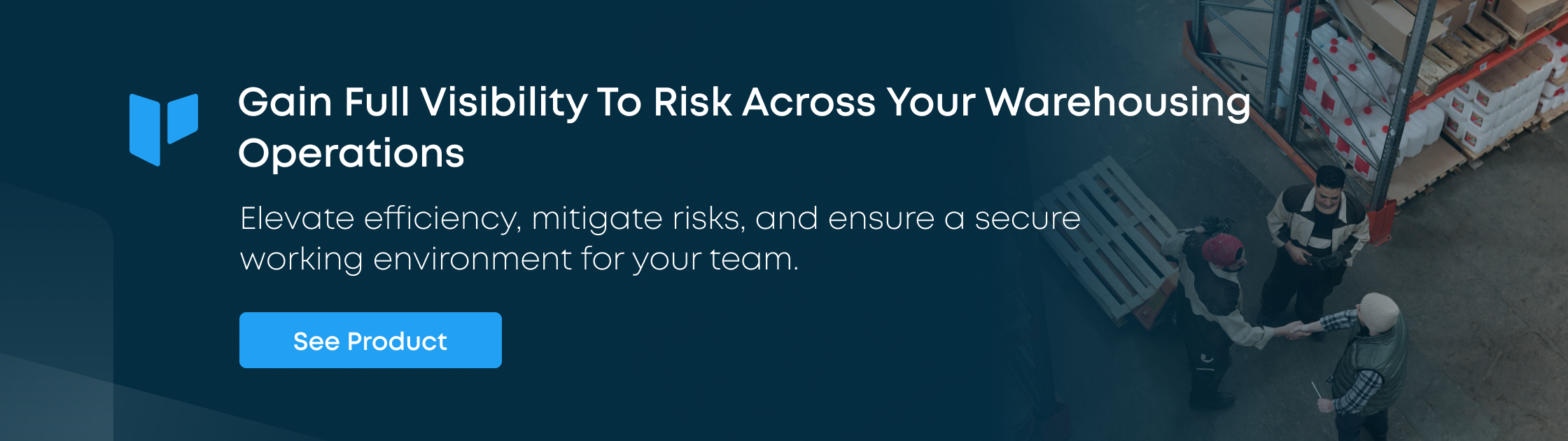
What you’ll learn:
- We start by describing AI, and then get into the detail of understanding your safety and health challenges in warehouses.
- We’ll touch on how warehouse safety technology is advancing rapidly, offering companies innovative ways to reduce risks and enhance safety measures across their operations.
- We’lll discuss how AI can provide extra layers of data control and real-time monitoring, helping you to identify the underlying causes of workplace injuries for each of the warehouse hazards considered.
- We’ll explain how to develop an AI model, using forklift truck management as an example.
- Having explained the use cases, if you are interested in the theory, the guide explains how monitoring with AI can add an extra slice to your ‘Heinrich’ triangle, providing more opportunities to fix problems before accidents occur.
- As we know there can be a lot of concern about the ethics of how AI is used, we have a section covering data privacy and the control of AI systems.
We’ve included a case study to show how one UK retailer applied AI-supported computer vision successfully, and the guide finishes by explaining the five steps you need to follow to move warehouse safety management to the next level.
What do we mean by ‘AI’?
Perceptions of AI have changed dramatically in recent times. For many years, the popular imagination of AI related to androids with human capabilities, so similar to real people that it would be hard to tell them apart. Or to massive computers, used to fight wars, or with an intent to overcome humanity.
Chat GPT and similar generative AI models have changed perceptions of AI – but not necessarily towards something more accurate or encompassing as AI has to offer. In this paper we will look at a broader spectrum of what AI can do, with a particular focus on health and safety in warehousing and logistics.
How does AI work?
Traditional software – like the database you might use to record orders, or the workflow system you use to track goods – consists of a set of instructions, written by programmers. When you use it, you are selecting from pre-defined rules. So you can select orders that are late, or shipments going to a stated location because a programmer predicted that’s what you might want to know.
With AI, new rules and instructions can be devised to do new tasks that the programmers never considered. It’s narrow AI, because unlike the AI of fiction, it only works within a specific field. A chess programme plays chess, and learns new ways of playing chess better.
Improving warehouse safety with AI-powered analytics
Data analytic tools are designed to look for patterns and meaning in numbers, which could be collected manually through reporting and observation, or via other tools such as sensors.
Computer vision can spot patterns in an image, and can learn to combine different types of image recognition to identify behaviors or situations. In a warehouse, computer vision can be trained to spot when a forklift truck is in a pedestrian zone, or when a pedestrian is in a vehicle zone. But it can’t play chess.
What is computer vision?
Initial attempts at teaching computers to recognise images were based on being able to define those images. So a cube has 12 edges, six square faces and eight corners; a regular pyramid has 8 edges, one square face and four triangular faces, and five corners.
But it was difficult to write an algorithm that could distinguish one shape from another at any angle, and in any lighting condition, or when one object was behind another. It’s even harder to define people, vehicles, racking and conveyor belts with algorithms.
Using AI-powered safety systems for warehouse operations
A key advance in visual AI has come through machine learning. Instead of having to programme the rules needed to recognise an object, the AI could be shown lots of examples of that object, and it would devise its own rules.
Do a Google image search for ‘forklift truck’ and you’ll see how easy it is to find images that can be used to teach AI to recognise forklift trucks of different shapes, sizes, colors and angles.

Warehouse safety challenges and AI solutions
In the UK workplace fatalities have reduced significantly in past decades. Although construction still leads the league table of fatalities, thirteen workers died in accidents in UK warehouses in the five years from 2017/18 to 2021/22. Over the same period an average of over 5000 workers per year suffered reportable injuries in UK warehouses.
Common warehouse hazards leading to injuries and fatalities
Some of the most common causes of injury and death across all industries will be familiar to those in charge of warehouse safety. In particular, 127 UK workers died after being hit by a vehicle across a five-year period to March 2022, and over 95,000 workers suffered reportable injuries from slips, trips or falls on the same level in the same period of time.
Warehouse safety statistics in the USA
Recent statistics in the USA continue to highlight significant safety challenges in the warehousing sector. According to data from the U.S. Department of Labor's Occupational Safety and Health Administration (OSHA), the average fatal injury rate for workers across all industries in 2023 was approximately 3.5 deaths per 100,000 workers.
The warehousing and storage sector experienced a higher rate, with 4.4 deaths per 100,000 workers, indicating that warehousing remains a particularly hazardous industry.
For the broader category of material moving workers, which includes roles such as packers and drivers in warehouses, the leading cause of fatalities continues to be incidents involving vehicles.
Other significant causes of injury include contact with objects and equipment, exposure to harmful substances or environments, and slips, trips, and falls. These trends underscore the persistent safety risks faced by workers in these roles and the need for continued focus on improving workplace safety measures.
Why warehouse hazard identification and risk management are important
Reported accidents are often only the tip of the iceberg. Minor accidents and near misses happen every day in many workplaces without being reported. Even minor accidents and near misses result in loss of time, productivity and damage to equipment and stock. And they can be an indicator of more serious problems.
Proactive AI safety measures for warehouse safety monitoring
Where dangerous situations are allowed to continue, such as vehicles driving at speed near pedestrians, or unstable racking, the regulator can prosecute even if there hasn’t been an accident. Responding to prosecutions is expensive and will damage the reputation of an organization.
Integrating AI into warehouse safety management systems
Your first tool for improving warehouse safety is your risk assessment – AI hasn’t changed that! What systems do your risk assessments have in place to monitor hazard controls?
As technology improves, controls and monitoring systems that might not have been practicable before become affordable and cost-effective. The growing adoption of warehouse safety solutions is allowing companies to automate risk monitoring and streamline safety compliance, ensuring that critical hazards are effectively managed.
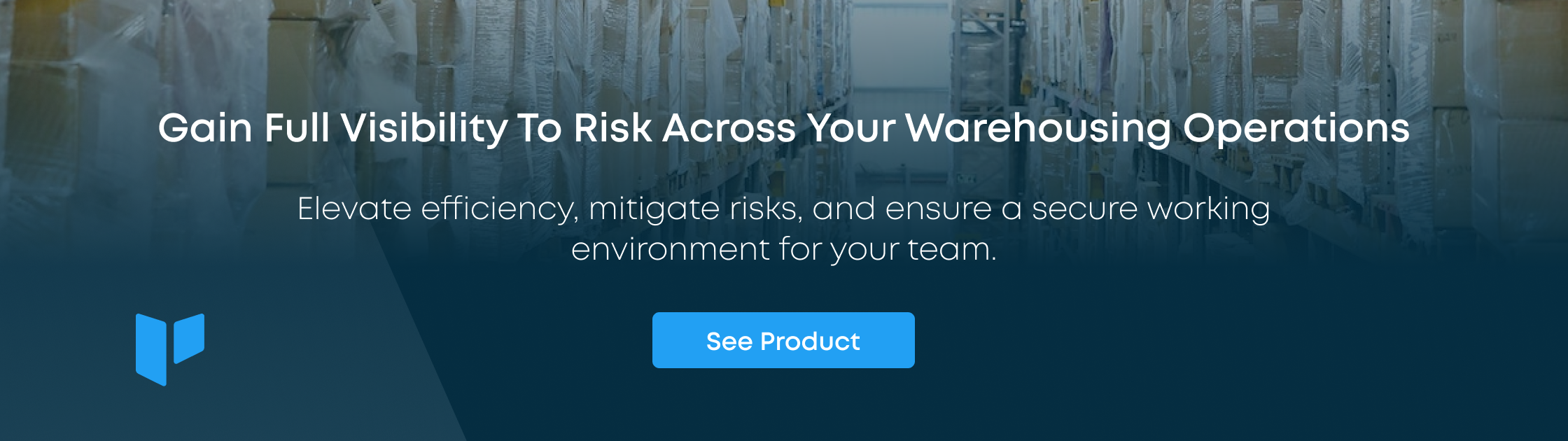
AI technologies for warehouse hazard monitoring
How can technologies such as AI help us with warehouse hazard monitoring? Here are some suggestions for each of the six hazard categories we identified in our warehouse risk assessment in Table 1:
Using AI for warehouse hazard detection and monitoring
The majority of reported injuries arise from slips and trips, many of which could be reduced by improved housekeeping and inventory management.
- When a delivery arrives at a busy warehouse it might not be possible to store the delivery immediately.
- Areas need to be defined for temporary storage, away from walkways.
- Computer vision in warehouse management can help by continuously monitoring these areas and identifying potential hazards, ensuring that walkways remain clear – and that pedestrians don’t take short-cuts through areas set aside for storage.
Detecting slippery conditions is a harder task for computer vision, but it will be here soon. Computer vision can also detect those minor accidents, where someone falls but unless they are hurt badly, they don’t report.
Using blockchain technology for inventory tracking
Blockchain technology is still developing, but in the future might provide some help to manage this hazard. Blockchain enables all your inventory to be tracked more accurately, making it easier to plan for deliveries and collections, and to be sure of the location of goods within your warehouse.
Objects fall from racking
Unstable items on racking can fall onto people, causing injuries. This can happen if racking isn’t stacked properly, or if objects are disturbed when a lift truck hits the racking. Objects might not fall immediately, but the collision leaves them poised to fall the next time someone else accesses the racking. Although you might require drivers to report all collisions, they might forget, or the time delay before they report means that someone has already been injured. Computer vision can send you an alert as soon as it detects a possible racking hit, so that you can review the video and check the stability of the stored objects.
Implementing warehouse safety technology for racking safety
Some stock control systems can support correct stacking, for example by requiring items to be scanned using QR codes on both the package and the shelf. Provided the data about the package is accurate, this can ensure that items are stored on the correct shelf according to weight and size. In the future, the Internet of Things (IoT) might provide shelves that assess their own loading, and send you an alert if they ‘think’ they are overloaded!
People fall from height
The ideal is to eliminate work at height in a warehouse. For example, rotating racking systems (also known as carousels) allows each shelf to be adjusted to waist height for loading and unloading.
Warehouse hazard identification at heights
Other systems use vertical conveyors or stacker cranes to access higher shelves. These technologies might be practical for some areas, but in most cases it’s too expensive to completely refit a warehouse to eliminate all work at height.
Climbing onto a vehicle to adjust a load, if you are in a position from which you can fall, also counts as work at height.
Computer vision can spot activity in areas where there should be no work at height – such as a rack or vehicle which should only be accessed using a forklift truck. But it can also help with monitoring how people work at height.
One common work at height accident occurs when people try to reach too far from a ladder or other access equipment, rather than descending and moving it. Computer vision can now detect when someone reaches beyond a safe limit.
Loads fall from or on vehicles
Segregation of vehicles and people will reduce the severity of the outcome when a load falls from a vehicle, or topples over inside a vehicle. But if a badly managed load causes a forklift truck (FLT) to overturn, the driver is likely to be harmed. Forklifts are more likely to overturn if driven with a raised load or turning on an incline. Routes should be designed to avoid the need to turn on an incline, and computer vision can be used to check that these routes are being used. Computer vision can also spot when an FLT is moving with a raised load instead of a lowered load.
Computer vision for forklift and pedestrian safety
Routes should be designed to avoid the need to turn on an incline, and computer vision can be used to check that these routes are being used. Computer vision can also spot when an FLT is moving with a raised load instead of a lowered load.
Manual handling - Reducing workplace injuries with AI
Manual handling controls should always start with the design of the work environment to eliminate or reduce manual handling, for example using pneumatic arms. Once you’ve done that, how do you make sure it's working?
Monitoring employee safety with AI-powered technology
Workers don’t always complain about handling tasks which cause aches and pains. This can be because of a macho culture, where no one wants to admit a perceived weakness. Or it can be from the experience that ‘nothing will change.’
Wearable solutions to this have been around for a while, but the most accurate ones require the worker to have sticky pads attached to their skin to detect muscle activation. While this can be useful for a limited trial to assess new work methods, it is unlikely to be acceptable to most workers in the long term.
Using computer vision to identify where people are adopting a poor posture is non-invasive, as it can use existing CCTV (closed circuit television) cameras. The findings can help you to identify where environment or work design changes are needed to support improved manual handling.
Vehicle movements
The majority of fatalities occur when people are hit by vehicles. Forklift trucks (FLTs) are normally speed-limited, but some accidents occur because the speed limiter has failed – or been tampered with.
Real-time monitoring for warehouse vehicle safety
Some FLTs are equipped with software that can read settings and send messages to a management dashboard about the speed settings.
But if you don’t want to replace your FLT fleet, computer vision provides a straightforward way to monitor the speed of existing vehicles – and visiting contractor vehicles, provided there are CCTV cameras in the right place.
Segregation of vehicles and pedestrians is another key control, and computer vision can spot when pedestrians and vehicles stray into each other’s areas.
Although high-visibility clothing is a weaker measure than segregation, sometimes people need to approach vehicles whether as a driver, or to assist. Computer vision can spot when pedestrians aren’t wearing high-visibility clothing in areas where it is a requirement.
Storage of hazardous substances - Improving warehouse safety compliance
Minimizing and storing substances correctly is a key feature of both your fire management system and your control of substances hazardous to health.
Ensuring compliance with warehouse health and safety requirements
Inventory control systems, where items are scanned in and out of each location, provide one way of checking how much of a substance you have, and that it is kept in the correct place. If there is a fire, the fire service needs to know the location of pressurized cylinders and flammables, so that information must be available on a mobile device.
When there is an emergency, everyone needs to know what to do quickly. That means practicing with spill kits for any hazardous substances you store or handle, and regular fire drills. In between, computer vision provides an extra set of eyes to check that exit routes are kept clear and that where internal fire doors need to be kept closed, they are shut.
Where the residual risk from handling hazardous substances, for example, when cleaning requires people to wear protective gloves or goggles as PPE, computer vision provides another way of monitoring whether this is happening.
Improving warehouse health and safety with AI
With all these examples, you can see how computer vision can provide you with an extra set of eyes – it’s like having a spare health and safety advisor who can watch what’s happening from several places at once, and then draws your attention to any incidents of concern.
AI workplace safety solutions like Protex go beyond traditional safety monitoring by offering proactive risk detection and real-time incident reporting, helping organizations maintain safer environments while boosting overall efficiency.

An example of AI model development
Imagine you have a system that can detect a forklift truck (FLT). But you don’t want it to create an alert every time it sees an FLT – it needs to know something about the context of use. Are there zones it should drive in, and zones it should avoid? Does the direction of movement matter, for example in a one-way system?
- Using computer vision for forklift and pedestrian safety
Using the CCTV feed of your workplace, you can ‘draw’ exclusion zones directly onto the image on your screen. You can mask out those areas where you need to know if the computer vision detects a vehicle (and which types of vehicles you’re interested in). And you can map separate areas where you need to know if pedestrians are walking.
- Adding AI-powered safety protocols for PPE detection
Once you have this working, you might decide to add more sophistication to the model. People can walk in some locations, for example, but only when wearing high-visibility clothing. If they are not, you want the model to detect this as an anomaly, and alert you.
- Validating and optimizing AI models for workplace safety
Model development works best if you start small, train the model and check that it is working as you intend before adding further functions. Validate by reviewing the anomalies identified and provide feedback as to which are correct.
- Fine-tuning AI models for safety gear compliance
For example, you might have different colors of high-visibility clothing in your organization for different roles. The model might start by recognising yellow high-vis as acceptable, but not accepting pink high-vis. Over time, you can optimize the model to recognise both.
Continuous improvement in AI warehouse safety management
OHS professionals will already be aware of the importance of collecting information about near misses (that is, events in which no injury occurs).
If you can see patterns from near misses and use these to enhance safety, you hope you can improve accident prevention measures. If you know that people are crossing the vehicle route regularly or that vehicles are speeding, you can find out why, and change what is causing this behavior.
Leveraging AI safety systems for better near-miss reporting
The principle is based on a triangle model, first used by Heinrich to represent different severities of insurance claims. The ratios between layers will vary by accident kind and industry, but the principle remains true: near misses give you more opportunities to learn and to find improvements than major accidents.
However, you probably also know how many near misses go unreported. Reporting systems can be time consuming, or people don’t want to be seen to be ‘telling tales.’ AI-supported monitoring systems such as computer vision, can add an extra layer to that triangle, as shown in Figure 1.
Data privacy and the control of AI systems
One fear that pervades all safety data collection and processing technology, whether based on traditional databases or AI-driven, is what happens with the data. Who can see it? Where might it all go wrong if too much power is given to the technology?
Managing data privacy and limiting the power given to the technology relies on two key aspects – the care taken by your provider, and how you and your organization use the data.
How to Choose a secure AI safety technology provider
If you use ChatGPT you should notice this warning each time you start a session:
Don’t share sensitive info: Chat history may be reviewed or used to improve our services.
So you shouldn’t, for example, give ChatGPT (or Bard or any other free platform) internal company data and ask it to analyze it for you. Don’t give it an accident report and ask it to re-write it. If you do, you are giving someone else the right to use your information in whatever way they see fit.
If you’re buying an AI product to support safety management you need to know your information and data is only for your organization to use, and that no one, not even someone working for your supplier, can access that information.
Using edge computing for AI safety solutions
ProtexAI achieves this using edge computing for its computer vision system. This means that your live CCTV is streamed via a managed ethernet connection from the CCTV network to an ‘edge’ device.
The edge device sits entirely within your system, reviews the video feeds and identifies the hazards you define. ProtexAI adds an extra level of security here, in that faces are blurred, and the video clips are encrypted before they are forwarded to the management system in the cloud.
Securing warehouse safety systems and CCTV networks
Computer vision can’t fix a leaky CCTV system, so check that your existing CCTV arrangements are secure. Best practice is that the camera network is physically separate from the corporate network.
As well as software controls over access to live camera streams, such as user authentication on login, remember the physical controls, such as preventing unauthorized access to the CCTV viewing room.
Review and update existing data protection impact assessments (DPIA) for your CCTV system when you add computer vision. If a DPIA isn’t a legal requirement in your location, it’s still good practice to do one, and can help to get workers engaged in the project.
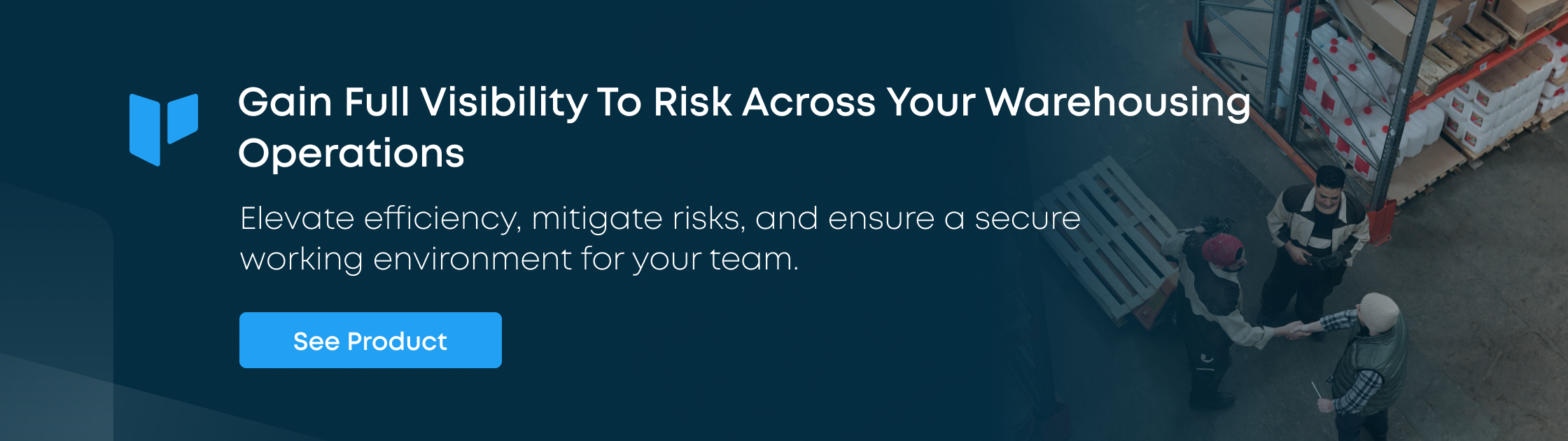
5 best practices to implement for AI adoption
Similar ethical principles for AI adoption have been proposed in the UK in the pro-innovation approach to AI regulation, in the EU AI Act, and in the Blueprint for an AI Bill of Rights in the USA. The application of these principles to warehouse hazard monitoring can be summarized in these five headings:
- Be transparent with your workers
Make it clear to people where CCTV cameras are located, for example by displaying signs. Explain where cameras are and how they are used during induction training, and when anything changes. Tell workers who will be able to watch the live stream, and how any recordings will be used.
While in most cases recordings will only be seen by the safety team, you need to let workers know when they might be shared more widely. For example, if there is an accident and a regulator is involved in an investigation, you might have to identify people.
When you introduce computer vision to an existing CCTV system through the use of AI cameras, you need to tell people when still images or film clips might be captured, who will see these and how they can be used. The most transparent approach would be to show examples of the type of information collected to your staff.
- Be specific (about what you want to use it for)
If you have existing CCTV cameras, you should already have considered the balance between the legitimate interests of the organization and the privacy rights of individuals. Adding computer vision changes the purpose of the cameras, and might impact the balance.
Your aim might be to identify safety concerns with the working environment (such as lighting or layout). Or you might be looking for examples of behavior to identify training or coaching needs.
If later you use the technology to compare how long different individuals spend on a task, or as evidence in a disciplinary case, you will be breaching this principle - and the trust of your workforce.
- Collect and keep only what you need
Information collected should be limited to what is necessary for the task. If the aim is to see how many people are too close to vehicles at a location within a shift, you don’t need the individuals to be identifiable.
Computer vision systems that blur the faces of people within the video clips will help you to meet this principle. The EU Act and some state-specific laws in the USA are likely to prohibit facial recognition and other biometric identification systems in public spaces, so check what applies to your workplace.
There should be a written retention policy for images. Your organization needs to be able to justify how long video is kept. For example, a month is probably long enough for a crime to be detected. If there is an accident in the workplace, relevant CCTV images can be kept for longer if it might be needed for an investigation.
- Human oversight
To keep AI in check we must keep the human in the loop. We don’t want AI systems that trawl through workers' emails or track every key press and send out termination notices if they think people aren’t working hard enough.
With computer vision, we don’t leave it to the AI to make the final decision as to whether a dangerous behavior occurred, or a dangerous situation arose.
AI-supported computer vision narrows down the hours of video that you would otherwise need to review – but the final decision on how to interpret the video, and what action to take, rests with a human.
The person with that oversight has a responsibility to use it fairly. For example, be careful if assigning labels to film clips – describe what you can see, not what you think. As an example, label a clip as “worker appears to drop a load” not as “careless worker”.
- Fairness
In the US AI Bill of Rights this is called ‘algorithmic discrimination protections’, while the EU Act refers to ‘diversity, non-discrimination and fairness’. Some of the steps we’ve already described contribute to fairness – a transparent system, with a human in the loop, allows unfairness to be challenged.
Discrimination can arise because of the data the AI models are trained on. For example, if most of your workers are men, make sure your model will also recognise – and protect – women.
Case study: AI-powered safety solutions in warehouses
The safety team at Marks & Spencer identified specific problem areas using computer vision, and targeted safety conversations in those areas.
In one location there was a 40% reduction in unsafe events in just one week. After three months, the number of unsafe events had dropped to just 20% of the initial measurement.
There is still room for improvement, as new workers and agency workers learn to work the M&S way. Existing workers see the AI not as a spy on the wall, but as a means of helping the safety team to keep them safe. You can read a more detailed account of their experience here.
5 Steps to implement AI in warehouse safety management systems
So what next? These steps should help you move your warehouse safety management to the next level
- Prioritize with purpose
It’s tempting to go to an exhibition, see something exciting, and adopt it. But start with the basics. Compare your risk assessments with Box 1. Yours will be more detailed, but is there anything omitted? Which hazards are you managing well – and where do you need more support?
If controls in your risk assessments state ‘the worker will not overload the shelf’ or ‘vehicles and pedestrians are segregated’ how are these controls monitored? If training is a control, do you have evidence that people apply their learning once they leave the training room?
Audits, accident and incident reports, inspection records, and safety observations will help you to identify target hazards to prioritize.
- Define the standards for relevant activities
For each of the hazards prioritized, what are the goals for the activities where they occur? You might need to refer to documents, such as safe operating procedures and method statements, which describe how tasks need to be done, who can do it, and what tools and equipment are needed.
Ask people doing the tasks about their understanding of the goals. Your activity – goal mapping might look like this:
Activity Goals
- Drive a forklift truck from the loading bay to a storage bay in the warehouse
- Drive at 10 km/h or slower
- Drive within vehicle zone
- Maintain greater distance from pedestrians when reversing
- Stack items on warehouse shelving
- Stack items on the correct shelf for the weight and size
- Don’t stack any shelf more than two boxes high
- Any odd-shaped items should be in boxes
- Collect stock from the warehouse to deliver to the production area
- Use a trolley when load is heavy or awkward
- Walk within pedestrian zone
Investing time to listen to your workers is an essential step in any change. Workers might suggest simple solutions without the aid of advanced AI technology! But other problems might be more stubborn, so move to the next step.
- Determine the best technology to achieve the goal
It’s better to manage two or three goals well, than to manage a dozen badly. Balance the risk benefit of achieving a goal with the cost in time, effort and money.
Checking that vehicles are driving within the speed limit might reduce the risk more than checking vehicles are in the right zone; using computer vision to check that people aren’t over-reaching might be easier to achieve than to check that shelves are stacked correctly.
- Run a pilot
Try the new technology in a limited area. Keep the worker representatives involved, as they will help you to see whether anything needs to be changed before you expand the programme to other parts of the workforce, and to meet other goals.
Use the pilot as an opportunity to discover what makes work rewarding to people. Listen to their challenges, and understand what will make it easier for them to manage warehouse hazards.
- Keep it going
Giving feedback at team level reinforces a culture where people support each other to do things safely. Tell a team that they are sticking to pedestrian zones 80% of the time, and you would like them to get a higher score next month.
Once a team achieves their goal for a whole month, continue to encourage and reinforce the behaviors. Make sure that doing the right thing continues to be the best option.

Additional references to explore
OSHA standards applicable in warehousing at www.osha.gov/warehousing/standards-enforcement
HSE guidance on warehouses at www.hse.gov.uk/logistics/warehousing.htm
Warehousing and storage: A guide to health and safety (HSG 76) at www.hse.gov.uk/pubns/books/hsg76.htm - explains how UK regulations apply in a warehouse and suggests appropriate controls.